Unveiling the Secrets of Data: An Empirical Approach to Dimensionality Reduction and the Study of Patterns

In today's data-driven world, navigating the vast ocean of information can be a daunting task. Traditional data analysis methods often fall short when dealing with high-dimensional datasets, where the sheer volume of variables makes it challenging to identify meaningful patterns. Enter dimensionality reduction, a powerful technique that transforms high-dimensional data into a lower-dimensional space while preserving its key characteristics.
This comprehensive article presents an empirical approach to dimensionality reduction, empowering you with the knowledge to extract valuable insights from complex datasets. Delve into the intricacies of different dimensionality reduction algorithms, explore real-world applications, and discover the practical implications for data analysis and pattern recognition.
5 out of 5
Language | : | English |
File size | : | 7074 KB |
Text-to-Speech | : | Enabled |
Print length | : | 325 pages |
Lending | : | Enabled |
Dimensionality Reduction: A Journey from Complexity to Clarity
Dimensionality reduction aims to reduce the number of features in a dataset without sacrificing the information essential for modeling or analysis. This reduction process reveals the underlying structure of the data, making it easier to visualize, interpret, and uncover patterns.
Several dimensionality reduction algorithms exist, each with its strengths and weaknesses. Principal Component Analysis (PCA),Linear Discriminant Analysis (LDA),and t-SNE (t-Distributed Stochastic Neighbor Embedding) are among the most widely used techniques.
Principal Component Analysis (PCA)
PCA is a statistical technique that identifies the directions of maximum variance in the data. By projecting the data onto these principal components, it effectively reduces the dimensionality while capturing the most significant information. PCA is particularly useful for data visualization and feature extraction.
Linear Discriminant Analysis (LDA)
Unlike PCA, which focuses on maximizing variance, LDA aims to maximize the separation between different classes or groups in the data. This supervised dimensionality reduction technique is often employed in classification tasks, where the goal is to distinguish between distinct categories.
t-SNE (t-Distributed Stochastic Neighbor Embedding)
t-SNE is a non-linear dimensionality reduction technique that preserves local relationships in the data. It is particularly effective in visualizing high-dimensional data, as it captures the neighborhood structure and reveals complex patterns.
Applications of Dimensionality Reduction: Empowering Data-Driven Decisions
Dimensionality reduction has found widespread applications in various fields, including:
* Data Visualization: Reducing the dimensionality of high-dimensional data enables effective visualization and exploration, making it easier to identify patterns and relationships. * Feature Extraction: Dimensionality reduction algorithms can extract the most informative features from a dataset, reducing the dimensionality while preserving the key characteristics. This process enhances the efficiency and accuracy of machine learning models. * Pattern Recognition: By reducing the dimensionality of data, it becomes easier to identify clusters, outliers, and other patterns. This aids in anomaly detection, clustering, and other pattern recognition tasks. * Image Processing: Dimensionality reduction techniques are widely used in image processing to reduce the dimensionality of images while preserving their essential features. This allows for efficient storage, transmission, and analysis of images.
Practical Implications: Enhancing Data Analysis and Pattern Recognition
* Improved Model Performance: Dimensionality reduction can enhance the performance of machine learning models by reducing irrelevant noise and redundancies in the data. This leads to more accurate and efficient models. * Faster Processing: By reducing the dimensionality of data, algorithms can process and analyze it more quickly, saving time and computational resources. * Enhanced Interpretability: Reducing the dimensionality of data makes it easier to interpret and understand the underlying patterns, leading to better decision-making.
Dimensionality reduction is an invaluable tool for unlocking the potential of complex datasets. By empowering you with an empirical approach to dimensionality reduction, this article has provided a comprehensive understanding of the techniques, applications, and practical implications of this powerful data analysis method. Embrace the power of dimensionality reduction and unlock the hidden patterns within your data, driving informed decision-making and empowering transformative data-driven strategies.
5 out of 5
Language | : | English |
File size | : | 7074 KB |
Text-to-Speech | : | Enabled |
Print length | : | 325 pages |
Lending | : | Enabled |
Do you want to contribute by writing guest posts on this blog?
Please contact us and send us a resume of previous articles that you have written.
Book
Novel
Page
Chapter
Text
Story
Genre
Reader
Library
Paperback
E-book
Magazine
Newspaper
Paragraph
Sentence
Bookmark
Shelf
Glossary
Bibliography
Foreword
Preface
Synopsis
Annotation
Footnote
Manuscript
Scroll
Codex
Tome
Bestseller
Classics
Library card
Narrative
Biography
Autobiography
Memoir
Reference
Encyclopedia
Nick Constable
Maggie Ford
Stump Connolly
Thomas Clayton
Larry Sitsky
Mahendra Rai
Len Krisak
The Princeton Review
Shannon L Alder
Lucy Newlyn
Lori Spagna
Michael Cohen
Margo Jefferson
Latoya Johnson
Lynne Sharon Schwartz
Maria Flook
Lynda Mcdaniel
Lorand Gaspar
Richard Taruskin
Mac Mcshane
Light bulbAdvertise smarter! Our strategic ad space ensures maximum exposure. Reserve your spot today!
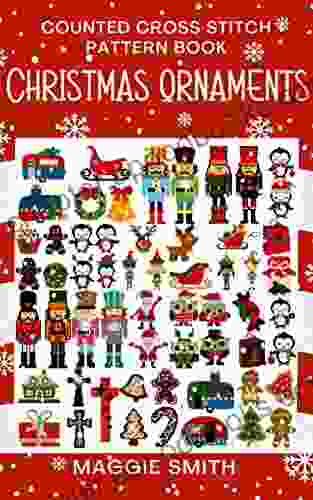

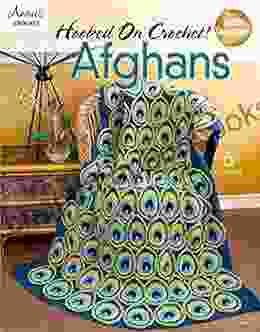

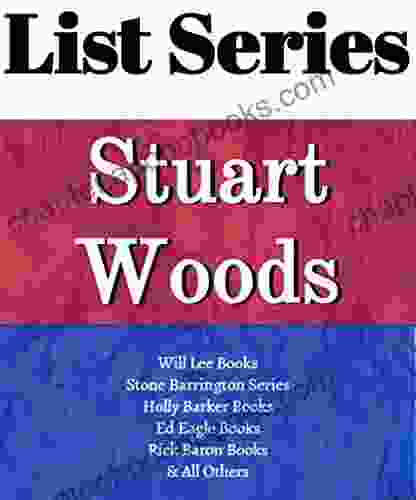

- Ian McEwanFollow ·6.4k
- Fernando BellFollow ·5.1k
- E.E. CummingsFollow ·13.1k
- Virginia WoolfFollow ·14.8k
- Sean TurnerFollow ·15.1k
- Levi PowellFollow ·12.7k
- Leo TolstoyFollow ·4.1k
- George BellFollow ·4.9k
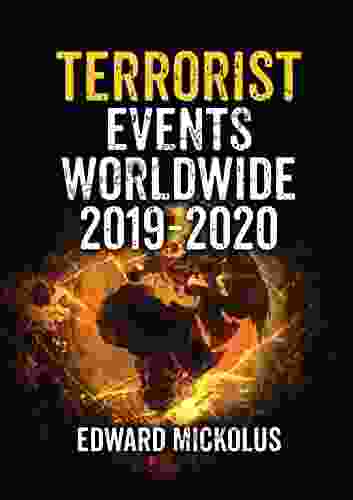

Terrorist Events Worldwide 2024: A Comprehensive Guide to...
Terrorism is a global threat that affects...
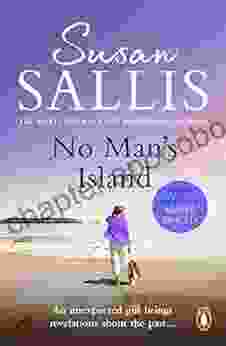

Beautifully Uplifting And Enchanting Novel Set In The...
Set in the beautiful West Country, this...
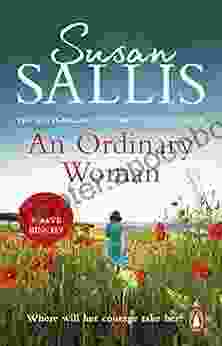

An Utterly Captivating and Uplifting Story of One Woman's...
Immerse yourself in an extraordinary...
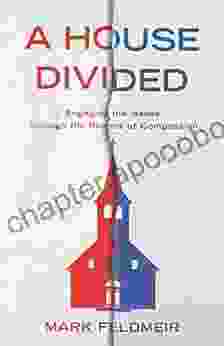

Engaging the Issues Through the Politics of Compassion
: The Power of...
5 out of 5
Language | : | English |
File size | : | 7074 KB |
Text-to-Speech | : | Enabled |
Print length | : | 325 pages |
Lending | : | Enabled |